Simple example of how Prediction \(\neq\) Inference¶
Let’s see the simplest example of how prediction task is different from inference task. (I borrowed this example from Susan Athey, the guru of ML+causal inference)
Suppose that you are tasked to measure the average height of musicians at the Vienna conservatory.
(– duh, can’t you find a better example, this one is used by every stat class ever? – I can, but let’s keep it simple).
Empowered by the knowledge of statistics, you randomly sample five people from the musicians and ask them their height.
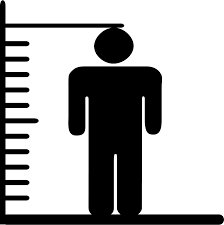
And you get the following five measures:
150cm, 160cm, 170cm, 180cm, 190cm
Estimate the mean¶
If you assume that height is distributed normally around some mean \(\mu\), then you would like to estimate \(\mu\) by running the following regression:
Given that the numbers in this example are so simple, can you “guesstimate” what you would get as the estimate for \(\mu\)?
You will find the answer on the next page, so you can check whether you were right.